
In simple random sampling, each potential random value within the probability distribution has an equal value of being selected. This can be useful for identifying which parameters have the largest impact on response standard deviation and which parameters could be changed to reduce the response PNC. In Monte Carlo sampling for simulation problems, random values are generated from a probability distribution deemed appropriate for a given scenario (uniform, poisson, exponential, etc.). Pareto plots of the contributions of each parameter to the response are also created. errors (LPE) and one of the favorite analysis tools, the Monte Carlo simulation using Latin hypercube sampling, are presented. Histogram plots of the responses, along with summaries of their sample statistics, will be created in a Monte Carlo report after all trials are run. This probability is called the Probability of Non-Compliance or PNC. If the response has a defined Upper Specification Limit (USL) or Lower Specification Limit (LSL) then a confidence interval on the probability of the response falling outside of these limits will be computed using the binomial statistics. Monte Carlo Analysis is the gold standard to which all other techniques are judged, and if computational expense is not a problem, then it will always be the preferred method. By then applying sample statistics a substantial amount of information can be derived about the response distribution – its location, spread and shape. Monte Carlo analysis approximates the output distribution by randomly generating single values for each of the input parameters, plugging these values into the model, and computing a value for the response.
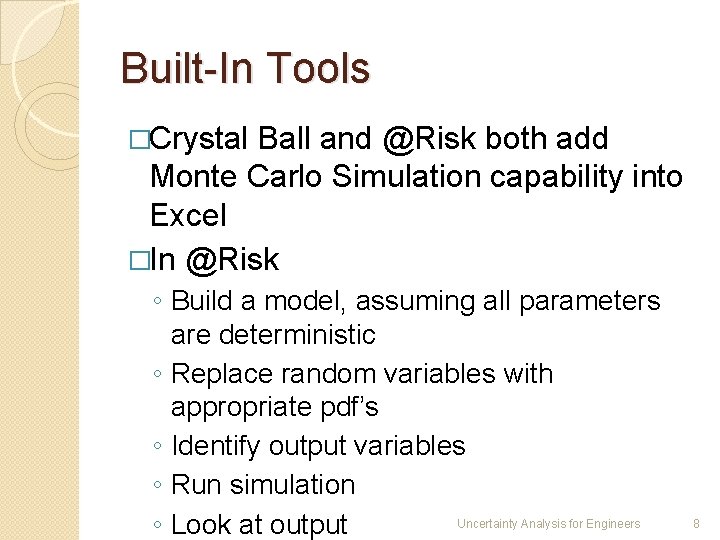
If the input parameters of a mathematical function have statistical variation, then the output response will also have variation.
